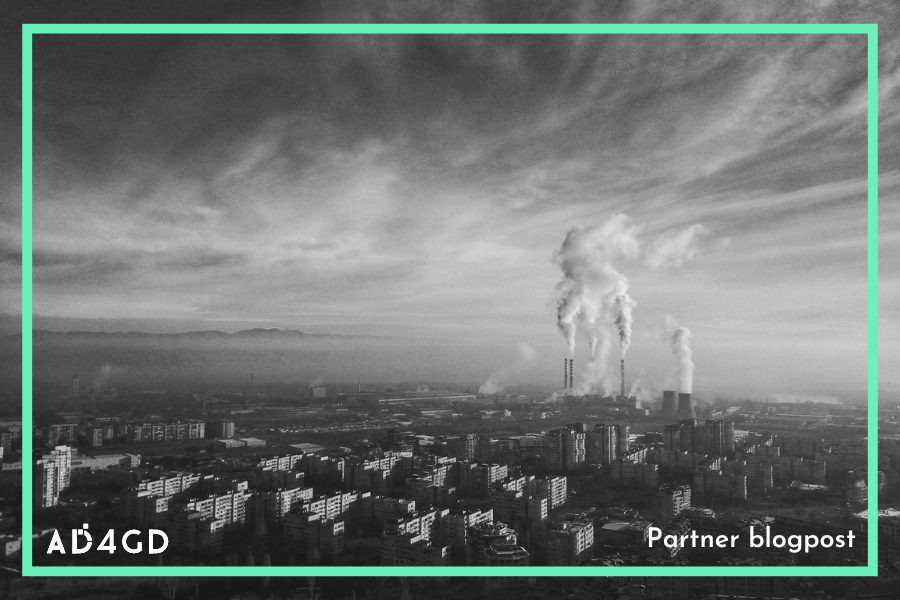
Air quality is vital for our health, with poor air quality leading to issues like asthma, heart disease, and even premature death. Despite ongoing mitigation strategies, many regions across Europe still exceed the thresholds recommended by WHO. Regions such as Northern Italy (particularly the Po Valley), Eastern Europe, and the Benelux are especially prone to poor air quality due to a unique combination of industrial and agricultural sources, compounded by topographic conditions that exacerbate pollution levels, as can be seen in the following picture.
Annual mean PM2.5 concentration across Europe for 2023 derived from analysis fields of the ensemble median of the CAMS regional air quality model. Data available via the Copernicus Atmosphere Data Store (link here).
Additionally, urban areas experience significant variability in air pollutant levels, causing traditional monitoring methods to often miss local pollution hotspots if not exactly placed in there. Conventional methods are also cost-intensive, making an accurate high-density air quality monitoring challenging. This is where IoT (Internet of Things) technology and low-cost sensors driven by citizen science come in, providing a more detailed and cost-effective solution for monitoring air pollution.
What are IoT and Low-Cost Sensors?
In air quality monitoring, IoT devices gather data from numerous points, providing a comprehensive picture of pollution levels. Low-cost sensors, which measure pollutants like particulate matter (PM2.5, PM10) and nitrogen dioxide (NO2), are affordable and can be widely deployed. By using many sensors , we can create detailed maps of air pollution, identifying highly pollutedion areas that might otherwise be missed. Additionally, these sensors can provide near real-time data, enabling quick responses to pollution spikes, which is crucial for issuing health advisories, particularly at the local and urban scales. Their affordability compared to traditional methods allows for broader monitoring coverage without incurring significant costs. This cost-effectiveness also makes them popular in citizen science projects.
The distribution networks of two such citizen science projects: Sensor.Community and PurpleAir is shown in the following figure. Both networks cover large parts of Central and Eastern Europe, illustrating the extensive reach and impact of these initiatives.
Distribution of IoT sensors across Europe.
Case Study: Air Quality Monitoring in Sofia, Bulgaria
An interesting example of how low-cost sensors can provide a holistic picture of air pollution within an urban area is Sofia, the capital of Bulgaria. Sofia is particularly prone to air pollution, due to its geographical location in a valley surrounded by mountains, which can trap pollutants. Additionally, heavy traffic emissions from older vehicles and widespread use of solid fuels for domestic heating exacerbate the problem.
However, the city also benefits from hundreds of low-cost sensors from Sensor.Community placed throughout the area. The data collected by these sensors enable us to create high-resolution maps of pollution levels, such as PM2.5, allowing us to clearly identify different pollution hotspots within the Sofia area.
Distribution of IoT stations in Sofia (left) and regridded PM2.5 concentration on 250x250m2 resolution (right). Colorshading indicates monthly mean PM2.5 concentration for December 2022.
Limitations of low-cost sensors
Low-cost sensors, while affordable and widely deployable, have several limitations. These include unknown measurement quality, uncertain reliability, or inconsistent availability. Additionally, they are often operated by untrained personnel, which can further affect data accuracy. To address these uncertainties, it is crucial to apply various statistical techniques (e.g. from machine learning), to correct and enhance the accuracy of the sensor data.
The challenge of heterogeneity
Low cost and IoT sensor data provides interesting challenges for data ingestion and standardization as it can be highly heterogenous in structure and semantic content.
For example, a measurement of air quality like PM2.5 may or may not come with information about the sensor that measured it, its unit of measure, its location on Earth, the physical device to which the sensor is attached, the period of time over which the measurement was taken, whether the measurement has undergone any transformations from the raw value such as humidity correction, and many more possibilities.
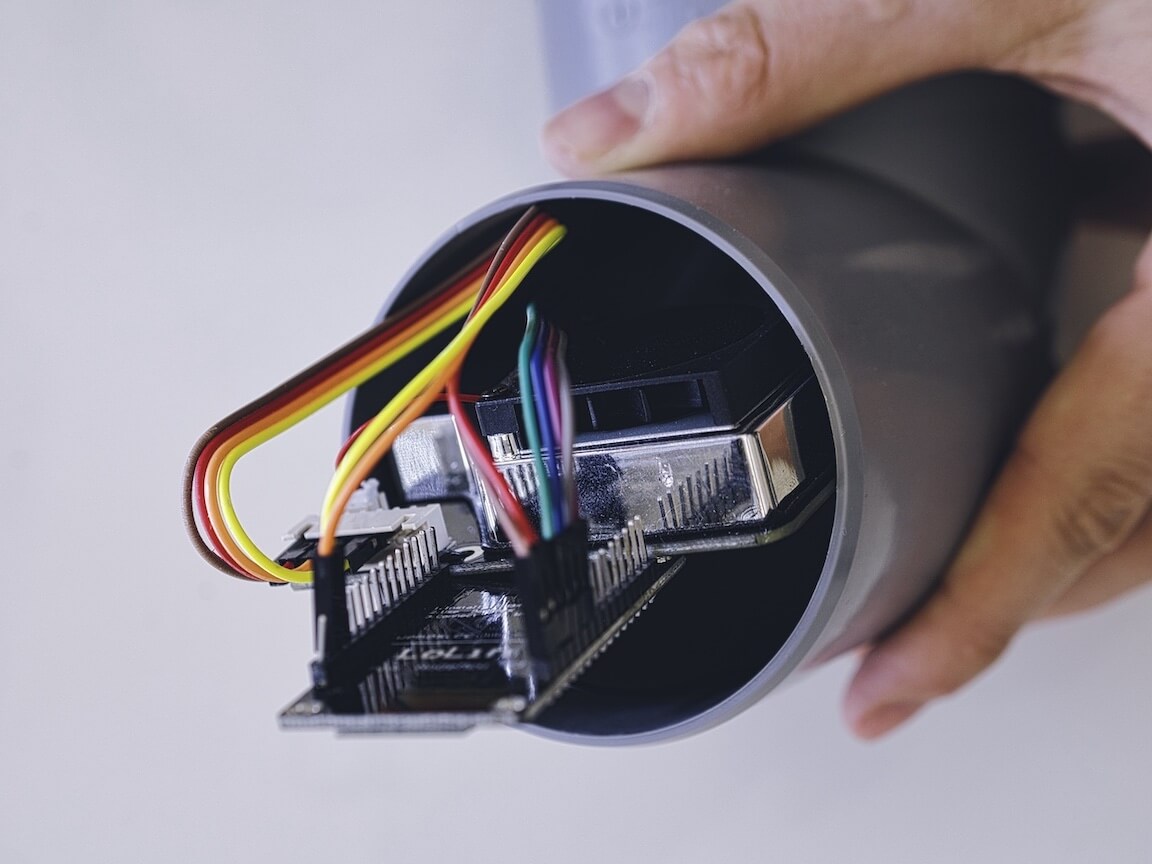
Low-cost air quality sensor. Image: Sensor.Community
Each actor in the chain between the raw measurement and the end user of the data makes decisions about how to interpret and represent this data and metadata and consequently the ecosystem of such data is highly diverse. This makes it difficult to easily access and compare data streams from different sources as the terms used and data structure may be very different.
AD4GD is exploring different ways to work with this heterogeneity problem. One example is through standardization and translation of vocabularies, which would allow one to automatically determine the relationship between terms used in different datasets. Another is to move towards greater adoption of OGC standards such as the SOSA model and the STAplus API.
Integrating IoT and low-cost sensors into air quality monitoring can significantly enhance how we track and respond to air pollution. While these novel measurements provide detailed information, it is crucial to address and account for their limitations. By doing so, we can fully leverage these innovative observations to improve our understanding of air pollution patterns, respond quickly to health risks, and ultimately ensure more effective protection of public health.